Online Analytics Portfolio – R markdown
This is the first post of several in which I will explore ways to build an online analytics portfolio. I think it’s beneficial to have demonstration-of-competency portfolio pieces online, and no better excuse to play with web dev than this.
I did most of my phd with R
, so first, I explore how to get R
online.
But why R
in a world where all the online tutorials seem to be using Python?
This blog post by Kevin Markham (from 2015!) summarizes the situation nicely:
Are you teaching “machine learning” or “statistical learning”?
The line between these two terms is blurry, but machine learning is concerned primarily with predictive accuracy over model interpretability, whereas statistical learning places a greater priority on interpretability and statistical inference. To some extent, R “assumes” that you are performing statistical learning and makes it easy to assess and diagnose your models. scikit-learn, by far the most popular machine learning package for Python, is more concerned with predictive accuracy. (For example, scikit-learn makes it very easy to tune and cross-validate your models and switch between different models, but makes it much harder than R to actually “examine” your models.) Thus, R is probably the better choice if you are teaching statistical learning, though Python also has a nice package for statistical modeling (Statsmodels) that duplicates some of R’s functionality.
So that explains my heavy use of R in my phd program – I used it for hypothesis testing for experimental designs, for which interpretability of models is key.
So I’ll show how to do both – how to get R stuff online and also Python, for more machine-learning-type stuff.
How to get R to Markdown
I use jekyll on github pages, which means that if I can get markdown, I can throw it in a post. Knitr and R-Studio makes that easy.
From rStudio (you should be using rStudio!), you just do File > New File > R markdown > From Template > GitHub Document
. This sets the output
at the top to be github_document
. Then when you knit the document, it converts your .Rmd into a .md
On an earlier blog post I demo’ed the “lendingclub” dataset. I’ll replicate a bit of that here.
A demo.Rmd
file may look like this:
---
title: "Demo .Rmd to .md"
output: github_document
---
```{r setup, include=FALSE}
knitr::opts_chunk$set(echo = TRUE)
```
```{r library, message=FALSE, warning=FALSE}
library(readr)
library(lsmeans)
my.data <- read_csv("https://raw.githubusercontent.com/deargle/deargle.github.io/master/assets/data/LendingClub_2007_2014_Cleaned_Reduced_NoTargetLeak.csv")
```
## Reference level with an intercept
If we fit a logistic regression model, by default, an intercept will be estimated. In R, by default,
the first level is used as the reference level.
```{r model}
m <- glm(loan_status ~ grade, family='binomial', data=my.data)
summary(m)
```
Knit
ing this file would produce a demo.md
file, in addition to an .html preview. For the above, that would look like this:
Demo .Rmd to .md
================
``` r
library(readr)
library(lsmeans)
my.data <- read_csv("https://raw.githubusercontent.com/deargle/deargle.github.io/master/assets/data/LendingClub_2007_2014_Cleaned_Reduced_NoTargetLeak.csv")
```
Reference level with an intercept
---------------------------------
If we fit a logistic regression model, by default, an intercept will be estimated. In R, by default, the first level is used as the reference level.
``` r
m <- glm(loan_status ~ grade, family='binomial', data=my.data)
summary(m)
```
##
## Call:
## glm(formula = loan_status ~ grade, family = "binomial", data = my.data)
##
## Deviance Residuals:
## Min 1Q Median 3Q Max
## -1.5829 -0.6222 -0.4769 -0.3523 2.3710
##
## Coefficients:
## Estimate Std. Error z value Pr(>|z|)
## (Intercept) -2.74868 0.09153 -30.030 < 2e-16 ***
## gradeB 0.63193 0.10614 5.954 2.62e-09 ***
## gradeC 1.20502 0.10544 11.429 < 2e-16 ***
## gradeD 1.53512 0.11264 13.629 < 2e-16 ***
## gradeE 1.83239 0.14778 12.399 < 2e-16 ***
## gradeF 1.95645 0.26251 7.453 9.14e-14 ***
## gradeG 3.66497 0.84165 4.355 1.33e-05 ***
## ---
## Signif. codes: 0 '***' 0.001 '**' 0.01 '*' 0.05 '.' 0.1 ' ' 1
##
## (Dispersion parameter for binomial family taken to be 1)
##
## Null deviance: 8070.2 on 9999 degrees of freedom
## Residual deviance: 7719.6 on 9993 degrees of freedom
## AIC: 7733.6
##
## Number of Fisher Scoring iterations: 5
Which, when copy-pasted into a post such as the one you’re reading right now and built on jekyll / github pages, would render as…:
Demo .Rmd to .md
library(readr)
library(lsmeans)
my.data <- read_csv("https://raw.githubusercontent.com/deargle/deargle.github.io/master/assets/data/LendingClub_2007_2014_Cleaned_Reduced_NoTargetLeak.csv")
Reference level with an intercept
If we fit a logistic regression model, by default, an intercept will be estimated. In R, by default, the first level is used as the reference level.
m <- glm(loan_status ~ grade, family='binomial', data=my.data)
summary(m)
##
## Call:
## glm(formula = loan_status ~ grade, family = "binomial", data = my.data)
##
## Deviance Residuals:
## Min 1Q Median 3Q Max
## -1.5829 -0.6222 -0.4769 -0.3523 2.3710
##
## Coefficients:
## Estimate Std. Error z value Pr(>|z|)
## (Intercept) -2.74868 0.09153 -30.030 < 2e-16 ***
## gradeB 0.63193 0.10614 5.954 2.62e-09 ***
## gradeC 1.20502 0.10544 11.429 < 2e-16 ***
## gradeD 1.53512 0.11264 13.629 < 2e-16 ***
## gradeE 1.83239 0.14778 12.399 < 2e-16 ***
## gradeF 1.95645 0.26251 7.453 9.14e-14 ***
## gradeG 3.66497 0.84165 4.355 1.33e-05 ***
## ---
## Signif. codes: 0 '***' 0.001 '**' 0.01 '*' 0.05 '.' 0.1 ' ' 1
##
## (Dispersion parameter for binomial family taken to be 1)
##
## Null deviance: 8070.2 on 9999 degrees of freedom
## Residual deviance: 7719.6 on 9993 degrees of freedom
## AIC: 7733.6
##
## Number of Fisher Scoring iterations: 5
Tada!
The markdown output can be saved as its own file, with a .md
extension. This file can be hosted anywhere that renders markdown,
such as a separate jekyll page, or even as a stand-alone github gist (example)
if you don’t want to do the blog thing.
In a future post, I’ll figure out how to render the above dynamically. I see that jupyter notebooks can render R, which means that sites like mybinder.org can render jupyter notebooks with R in them, but the flow from RStudio to jupyter notebooks is not yet clear to me.
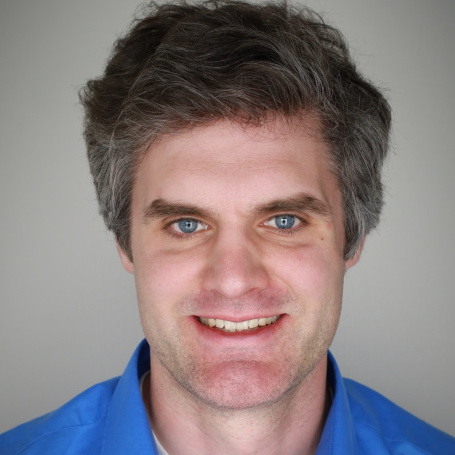
Dave Eargle is a Senior Consultant in Cybersecurity Assessment at Carve Systems. More about the author →
This page is open source. Please help improve it.
Edit